Progress in AI development to date has largely been through model-centric AI which focuses on AI systems' algorithmic aspects. In other words, it focuses on refining algorithms to improve performance, often relying on large, computationally intensive models. The perfect example is the evolution of GPT-3 with 175B parameters to GPT-4 with 1.7T parameters. However, as enterprises build ML models and applications, they quickly realize that the model-centric progress we’ve made to date does not come without its own set of challenges, like its reliance on top-of-the-line, supply-constrained GPUs. Moreover, as enterprises utilize the same underlying models, they realize that the only way to differentiate is to leverage some source of proprietary data. As a result, we continue to see a reinvigorated interest in data-centric AI, a discipline that focuses on data quality as opposed to model robustness, and solutions to help enable this transition.
The Limitations of Model-Centric AI
Model-centric AI dominated the early stages of AI development and has seen amazing progress over the years. Despite its advancements, this approach faces several challenges:
- Large Data Requirements: Effective model-centric AI typically needs large datasets, which can be challenging to acquire and process
- Reliance on High-End Hardware: Due to the large datasets, model-centric AI often requires expensive, high-end, supply-constrained GPUs, making it inaccessible for many organizations
- Overfitting Risks: Complex models can overfit to training data, reducing their effectiveness on real-world data and the types of input data
- Lack of Customization: Using generic models trained on public datasets leads to a lack of differentiation in AI solutions
The Advantages of Data-Centric AI
Data-centric AI represents a shift in focus to the quality, consistency, and relevance of the data used in training and operating AI models. Unlike model-centric AI, this approach prioritizes data optimization and curation as the key driver of AI performance. It involves meticulous data cleaning, annotation, and management processes, ensuring data accurately reflects the problem space and remains current. This method allows for simpler models, as the emphasis is on extracting maximum value from well-curated, contextually rich datasets. As a result, with data-centric AI, many of the challenges of model-centric AI are addressed through:
- Reduced Hardware Dependency: By focusing on data quality, there's less reliance on high-end GPUs
- Enhanced Model Generalization: Improved data quality leads to models that perform better on real-world data
- Heightened Differentiation: Customized AI solutions become possible through the use of proprietary, high-quality data
Ethical AI Practices: Better data management practices contribute to the development of fair and unbiased AI systems
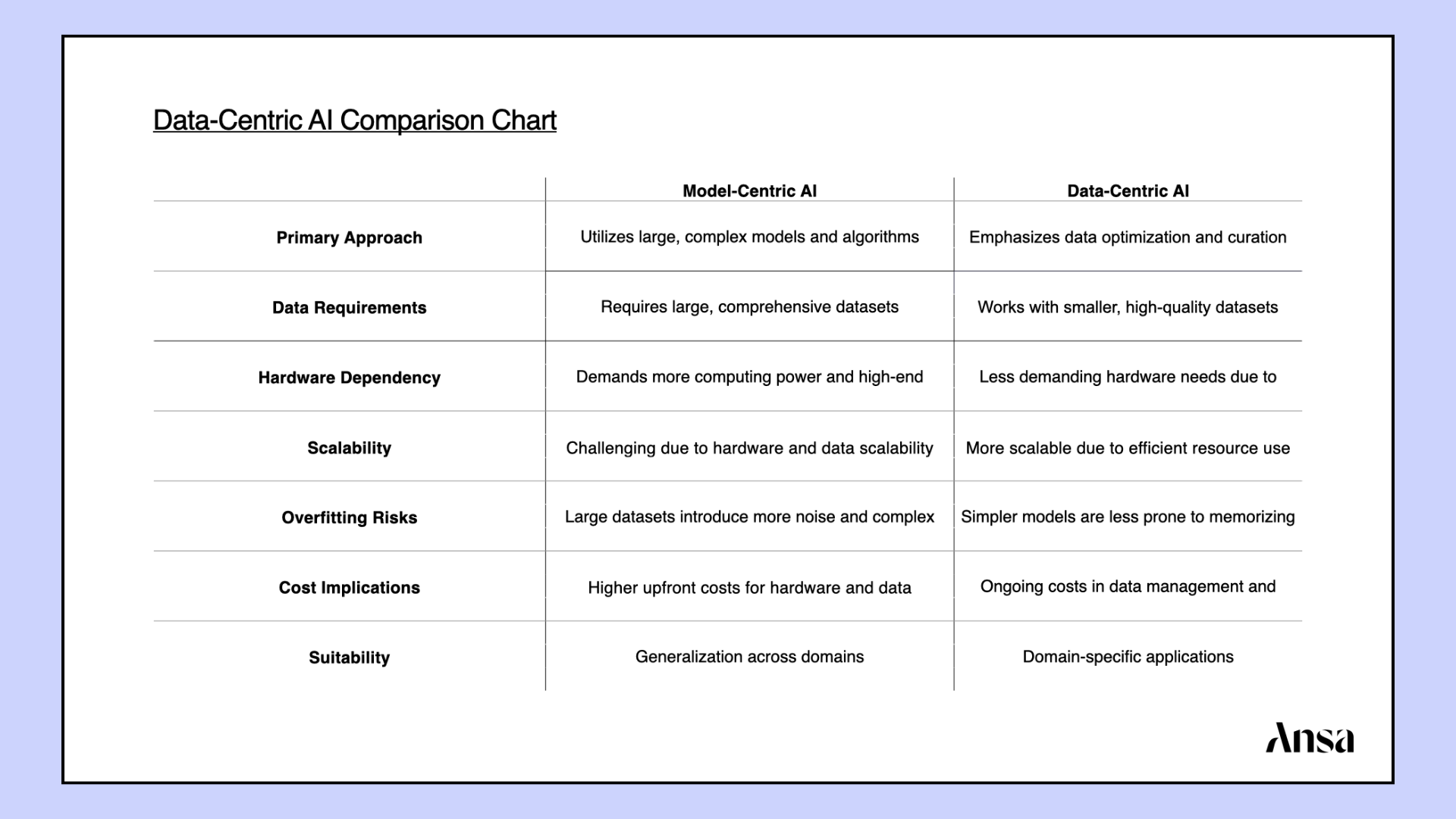
Future Outlook
Looking ahead, we see the importance and application of data-centric AI only continuing to increase. Every industry can benefit from domain-adapted AI knowledge, from healthcare diagnostics to industrial repairs. Given how prevalent IoT devices are becoming in many industries, the need for smaller models that can run with less computation resources only increases. Furthermore, as models continue to ingest more data, it is clear that greater emphasis will be placed on data-centric AI that focuses on privacy and security.
Overall, the industry has many compelling reasons to prioritize data challenges over model challenges. From tools helping enterprises leverage proprietary data to smaller, verticalized models, we’re excited by startups that are helping propel the industry towards data-centric AI and ultimately helping enterprises extract more value from their AI initiatives. If you’re building in the space, reach out to josh@ansa.co!